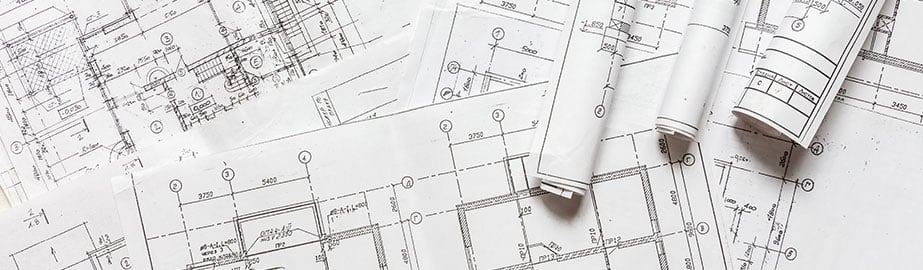
We’ve opined extensively on the topic of data—from vendor innovation to data architectures to data analytics. In many cases, we touch on broad trends and ideas on the evolution of data management, but it can be easy to lose the forest for the trees when it comes to applicability in a business setting. During the many years I have spent working on data technology, data office, and as a data product manager, I’ve noticed that despite new tools and processes, many investment managers still lack an effective data strategy. In this blog, I am going to take a step back and outline the key elements to building a holistic data strategy.
Oxford dictionary defines strategy as “a plan of action or policy designed to achieve a major or overall aim.” At its highest level, data strategy should help companies win. At the risk of oversimplifying things, there are three tenets to how this can be achieved:
First, data strategy should articulate how data will help achieve the overall business goals and outcomes.
Second, data strategy cannot exist on its own--it should be alongside business and digital transformation strategy.
Third, technology strategy must give support to data strategy.
Business strategy, data strategy, and technology strategy all should work together to provide the capabilities to win in digital. The very first step here is articulating the company’s vision statement for the data organization.
Step One: Articulating Data Strategy
A vision statement for a data organization might read: “create trusted high-quality data available to stakeholders on time to enable transparent data driven business decisions, investment decisions, and operational decisions to reduce risk, increase efficiency, and discover insights.”
Such a vision will move the organization towards data democratization (more on that in Creating A Democratized Data Organization, a recently published blog from Derek Pratt) and data literacy. Data literacy is a skill1; How many people in your business can interpret data from straightforward statistical operations such as correlations or judge averages and make decisions? How many managers can construct a business case based on concrete, accurate and relevant numbers? Data literacy is the ability of a business user to query the data, join data sets, visualize the data, generate insights, and finally be able to tell a story or make decisions with data.
With a vision statement articulated that propels more users toward data access and literacy, we can deduce capabilities needed. For this example, I’d define the organization’s needs as such:
- Data must be described, organized, and simplified with a data catalog and data dictionary, data lineage, data privacy attributes, active metadata (reference data and tags), visualization of data relationships between data domain, and data model
- Data must be quality checked and the organization must be able to see data quality metrics and rules, with the ability to document exceptions and other comments related to data quality
- Data must be secure and easy to access via a data marketplace for business intelligence and analytics using a simplified semantic layer with data level security controls, and anonymized data where needed to remove biases
These capabilities can then be achieved through effective data governance to act as a guardrail or control mechanism to make data fit for value generation.
Step Two: Tying Data Strategy to Business Strategy
A strong vision statement for data can fuel an organization’s data maturity, but without a tie-in to business strategy, this effort will be rudderless. A firm’s overall business strategy should inform any overarching data program—whether on a short or long-term horizon. Below are two examples of how this might work in practice.
If the business goal is support for upcoming ESG reporting regulation, then the main business goal is to make ESG metrics visible in the front office to support portfolio managers. In turn, data strategy goals would likely include fixed time to onboard the next new external ESG data vendor, a data dictionary for ESG data elements in a data catalog tool, and ESG data elements available in a data marketplace for the research team.
In another example, if reduction of operational risk events by 80% is the business goal, then the primary data goal might be to provide data to identify root cause of an operational error in a BI dashboard. With an effective data strategy, this entire data set could also be made available to train machine learning models that can be used to predict an operational error event. In addition, the data science team could access the same data in an internal data marketplace to analyze and find insights i.e. which operational process has the most risk.
While business strategy should always be striving toward maximizing revenue by generating business and investment insight, it’s key to understand where more granular goals lie. In these previous two examples, data strategy can be made more tactical and achievable by aligning with key business priorities.
Step Three: Technology to Support Data Strategy
To achieve an organization’s data strategy, governance process changes are typically needed which, in many cases, necessitates new levels of technology support. Historically, technology teams worked on data technology with help from the business in order to create a data warehouse or business intelligence reporting. When data governance was introduced, few technology organizations changed their process to enable seamless data governance. Many still maintain people-centric data governance models using Excel to track data dictionaries with little availability of data quality metrics or rules and no context information on where the data came from, where it is consumed, and how it is used.
To implement a technology-supported data strategy, a technology delivery process should be integrated into data governance. For most organizations, this will likely include the introduction of a new generation of tools such as metadata management platforms or DataOps platforms. With such functionality, business analysts can document the context of the data and data dictionary for the data consumers while data stewards are able to define data quality rules for data elements. At the same time, data architects can enable usage of data with relevant security to the end users--this integrated process of building a data pipeline is often referred as DataOps.
For an effective data strategy along with existing master data tools, ETL tools, data warehouse tools, tools that enable DataOps should be introduced to enable seamless data governance to integrate technology and data strategy.
Many asset managers have programs to modernize their data and analytics infrastructure and many may have some or all of the capabilities described here. Yet, in my experience, most managers are not yet able to generate maximum value from their data. Building an effective and holistic data strategy starts with the clear business goals, aligning these business goals to data and technology goals, and providing the tools to make data governance an enabler instead of an impediment. A holistic and well-defined strategy will enable your organization to make data driven business decisions, investment decisions, and operational decisions to reduce risk, increase efficiency, and uncover new insights.
1 "A Data and Analytics Leader's Guide to Data Literacy," Gartner, 2021
Comments